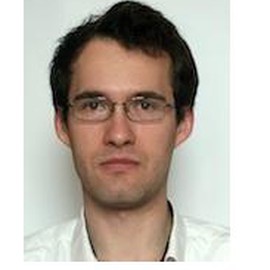
Mojmir Mutny
I am a postdoctoral researcher at ETH Zurich working at the intersection of Machine Learning and Optimal Experiment Design – addressing the search for the most informative experiments. I work in the research group of Andreas Krause. I am passionate about laboratory automation and protein design, and AI4Science revolution.
- Zurich
- ETH Zurich
- Google Scholar
- ORCID
- Github
- X (formerly Twitter)